Episode 71:
The potential of demand forecasting with artificial intelligence
See all episodes
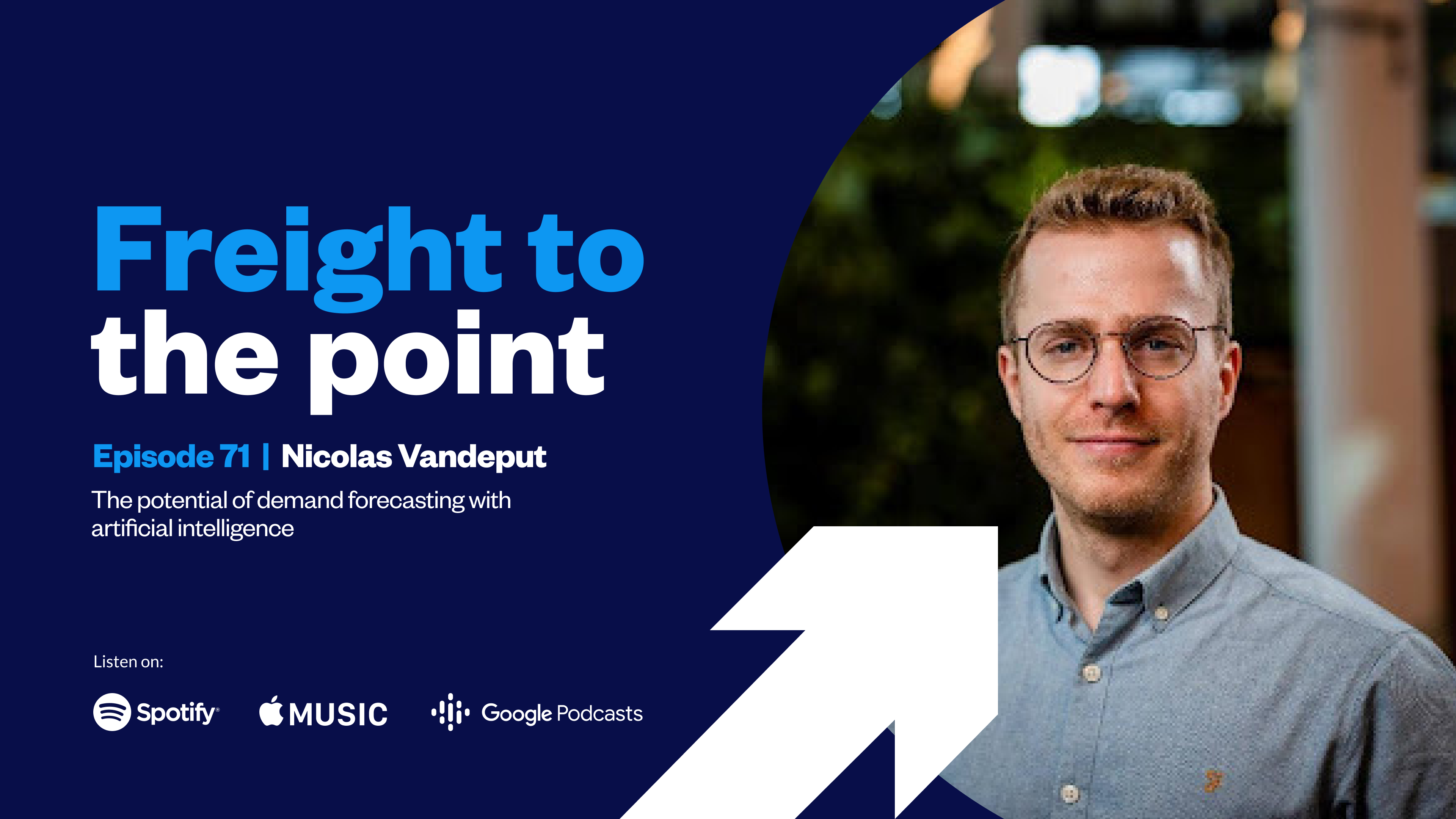
In the most recent instalment of Freight to the Point, Lucie Phillips, Zencargo’s Chief Operations Officer, engages in a discussion with Nicolas Vandeput, the CEO and Founder of SupChains, a company which specialises in demand forecasting and inventory optimisation. In this conversation, they delve into the significance of demand forecasting in supply chains and the potential it holds when combined with technology and machine learning.
They explore:
- The starting steps for any company who is looking at demand forecasting
- The KPIs that need to be put in place when it comes to forecasting
- What role technology and AI will play in evolving demand forecasting.
Nicolas Vandeput
Nicolas Vandeput is a supply chain data scientist specialising in demand forecasting and inventory optimisation. He founded his consultancy company, SupChains, in 2016; and SKU Science, an online platform for supply chain forecasting, in 2018. He enjoys discussing new models and how to apply them to business reality. Passionate about education, Nicolas is both an avid learner and teaches at universities. Since 2020, he has been teaching demand forecasting and inventory optimization to master students in CentraleSupelec, Paris, France. He published three books: Data Science for Supply Chain Forecasting in 2018 (second edition in 2021), Inventory Optimization: Models and Simulations in 2020, and Demand Forecasting Best Practices in 2023.
Resources
Episode 47: How should businesses approach inventory management?
Lucie Phillips:
Hello and welcome to another episode of Freight to the Point, a podcast by Zencargo. I’m Lucie Phillips, Chief Operations Officer at Zencargo. And, today, I’m thrilled to be joined by Nicolas Vandeput, CEO and Founder of SupChains. SupChains specializes in forecasting and inventory optimization, offering consultancy and training to its clients to support their supply chains. Together, we’re going to delve into the intricacies of demand forecasting and inventory management, exploring the challenges and opportunities that lie ahead. As AI continues to revolutionize industries, we will be uncovering its immense potential to elevate demand forecasting, and how it’s going to help businesses stay ahead of the curve. So very warm welcome to Nicolas. Would you like to give us a quick introduction to yourself?
Nicolas Vandeput:
Hello. Thank you, Lucie, for the opportunity to be here with you. Yeah, sure. Well, I always like to introduce myself with the same ID. I’m generally someone who’s passionate about inventory optimization and demand forecasting. And I’m only focusing on these two fields for the last… I think I’ve been doing that for the last six or seven years, and I just plan on doing that for the next 30 years or so. So yeah, that’s how I grow. And I’m trying to… So I have these two fields, and I’m trying to attack these two from different angles. I spend a lot of time creating content, articles, books. I’m also teaching that at multiple universities. I’m training professionals with this, and I’m also creating my own models. So the same concept like demand forecasting, I’m going to try to take it from many different point of views.
Lucie Phillips:
Great. Well, that sets us up nicely. It’d be good if you could maybe start off by giving our audience a bit of an overview of what demand forecasting is and how it plays a role in effective inventory management?
Nicolas Vandeput:
Yeah, sure. So the way I see demand forecasting, for me, it’s really like a supporting role, where your objective is not to make decisions, but it’s to basically get information, get insights about what’s lying ahead for your company. So, basically, you’re trying to predict the future so other people in your company can make decisions. It could be a decision related to inventory, like how much to produce, how much to buy, how much to ship. But it also can be decisions such as, “Should we hire new people? Should we open a warehouse and move a factory?” These kind of things. So the better you are at predicting the future, the better other people in your team, in your company, can make decisions.
And, for me, this difference between people who are, or processes who are, responsible to just get information about the future, versus processes and people who are responsible to make decisions, it’s quite important. Because what I see in many supply chains is that you get a corrupted process, where you have, at the same time, people pushing for some specific type of decision who are pushing the forecast in some specific direction. And we then see that the forecast is biased, in the sense that it could get overly optimistic or pessimistic, because people have incentive to push it in a specific direction.
Lucie Phillips:
I see. And so how do you then… Well, first of all, how do you assess which is the right forecast to be looking at? And how do you measure the accuracy of the demand forecast? And how do you even go about starting this? How do you get the data to make sure that it is accurate?
Nicolas Vandeput:
Yeah, so the first thing with a forecast is really to understand that you only do this really because you want to support other people, other teams, in making the best possible decision. So I think the first step is really, before even you try to assess, “Hey, is my forecast accurate? What kind of data I need?” It’s just to understand what kind of decision I’m supporting. And, for example, what kind of granularity of information these people would need. So, for example, let’s imagine you want to help the production manager of a factory to plan for what they should produce next week. So it means that you might have to have a weekly forecast per project, for example. And that’s already very important because a lot of companies don’t really do this step. And by default, they can, for example, measure accuracy per month, whereas the production facility and the logistic process works per day or weekly. So there is a discrepancy between what we are measuring, monthly accuracy, versus what we should be really doing, daily or weekly forecast.
So let’s imagine you took the time to discuss with the various teams you have to understand what they need to do and the granularity of decisions they’re making, then you can move on to tracking accuracy. Something that’s also critical, and I think some supply chains are still missing it, is when you forecast demand, you really have to forecast something I call the unconstrained demand from your clients. So in the sense that you really want to think, “Hey, how many products, and what type of products, and when do my clients want my products?” Rather than trying to forecast, “How much am I going to ship tomorrow?” Because how much are you going to ship, or produce, or sell tomorrow is very dependent on how much goods you have today. So it’s constrained by your current inventory.
But the forecast I’m advising to do, our clients in market oriented, it’s more a question like, “What does the market want?” And then it’s up to your team to deliver on this. So when we try to assess accuracy, we should really try only to do that based on the real requests from your client and the market, versus comparing your forecast to what you actually delivered, which might be impacted by any kind of shortage.
One last word on this, in terms of forecasting accuracy, and actually it gets very interesting and that’s why I love this subject so much, you really have to mathematically get a bit into the detail on how you assess the accuracy of a forecast. And it gets a bit technical. And, again, that’s why I love this subject so much because it’s not just about accuracy, but it’s also about being unbiased. So as I said earlier, you really need to ensure that, overall, your forecast over the midterm or long-term, they’re not overly optimistic or overly pessimistic. Like you’re always over forecasting by 10%, 15%, or always under forecasting by 10% or 15%. And usually if you do that, it means that somewhere in your process, someone or one team has a direct incentive to push the forecast in a specific direction. So you need at the same time to measure the accuracy and to ensure that you’re not biased.
Lucie Phillips:
That’s really interesting, so two questions on that. And you’re saying look at what the market wants, rather than what you think you just necessarily need to ship. How far out do you recommend people look when they’re looking at that market forecast in order to inform their decisions?
Nicolas Vandeput:
Well, it really depends on the type of supply chain lead times and decision you want to make. So, for example, right now, I’m working on a project where we want to look 28 days ahead. Because I’m working with a fashion retailer and, say, I have 150 stores, and we need to know from the central DC how much they should ship to this store. So when you do that, actually, just one or two weeks horizon is just good enough because you don’t need to plan shipment to your store six months in the future. Now, for some other industries, if you look, for example, at the pharma industry, they might want to have a one year and a half horizon because they need to give these forecast and their plan to the supplier for raw materials and so on. So depending on your industry, depending on the decision you want to take, you might have a very different horizon.
Again, for me, when I start a project with a client, with the supply chain, when I train people, I’m always starting with the question. Now, look, we only do these forecasts to support other processes in making the right decision. The question is, what kind of decision are we supporting? If it’s long-term investment and you have suppliers that have, I don’t know, a six-month lead time, then, for sure you need to have a very long forecast. But on the other side, if you just want to know what do you need to put in this drug that’s leaving tomorrow to replenish one of your stores, you might just need the one or two weeks forecast.
Lucie Phillips:
Okay. So it depends, obviously, on the industry, but it could vary. And you might want to do a slightly longer one, shorter one, but, again, depends on the seasonality of the market. That makes sense.
Nicolas Vandeput:
Exactly.
Lucie Phillips:
And so when you’re then also saying, the second part question I had there about don’t be too optimistic, don’t be too pessimistic, how do you advise your customers to essentially put KPIs or any kind of indicator in their processes so that they can closely monitor that type of accuracy? How do you spot where, say, one team is being more optimistic than another? How do you recommend they do that?
Nicolas Vandeput:
So, actually, there is a framework that exactly does just that. So the name of this framework is called forecast value added. And the concept is simple. And that’s why I love it so much because it’s simple so people understand it easily and we can implement it easily. The idea is really that once you start generating a forecast, so normally the process of generating a forecast starts with you have a model, statistical machine learning, maybe it’s just an Excel file, but you have a model creating a baseline. And then different teams, different individuals, will enrich that, while based on various insights. So maybe the sales team, maybe one of your demand planners, maybe, I don’t know. A lot of people could interact with your forecast and enrich it and change it over time.
Now, back in the days, let’s put it like that, you, as a manager, as a leader of this process, you would track the accuracy at the end of the road and say, “Well, overall with this baseline forecast and all the enrichment we did, this is the accuracy and the bias we achieved.” Now, the issue with that is you don’t really know who did what. You don’t really know if people were adding value, removing value, if people have been biased, you have no idea.
Now, with the forecast value added framework, the concept is very easy. We are going to track the accuracy achieved by every single person in the process. So we start with the software and we know, okay, once you get your baseline forecast out of this software, you get an accuracy of, let’s say, 60%. Okay, great. And then you have maybe a few demand planners reviewing that and they increase the accuracy to 65%. That’s amazing. That’s great. And then they give it to the sales team. The sales team change it, but the accuracy drops to maybe 62% now, and the bias that’s plus 5%.
Now, if you just do that one month or one week, you don’t really know where they’re lucky, unlucky, maybe that was just a bad month. But if you do this over time, after three, six months, and you see that consistently the same kind of team always over or under forecasts, then you know that, well, there is an issue with incentive. Because they tend to be, well, too optimistic or pessimistic. So, typically, what happens is maybe they get an incentive for beating the forecast. Maybe they say, “Well, if the forecast is 100 and I managed to sell 120, I get a bonus.” So, of course, they have a direct incentive to reduce the forecast.
It could be the other way around. Maybe it’s they’re thinking, “Okay, we always have shortages, so I cannot sell. So I’m going to increase the forecast so I’m sure that they get enough supply, and I’m able to sell to my clients. So I’m going to increase the forecast to 120.” Because people start to confuse the unconstrained demand forecast versus the supply plan, which are two different concepts. Again, if you don’t track the forecast value added of every team and individuals, there is no way you can really, basically assess who is adding value and who is not, which part of the process needs improvement. Now, as soon as you start measuring this person by person, or team by team, you can really start to go and have a discussion with these people because you can really identify who is biased.
Lucie Phillips:
So moving on to our second topic, let’s talk a bit more about technology and machine learning. So as we all know, AI has become such a huge topic in the industry. And we’d love to know how it’s played a role so far from your perspective, in terms of demand forecasting, inventory management? And maybe some examples of where you’ve seen it improve efficiency to date.
Nicolas Vandeput:
Yeah, sure. So I’m lucky enough to have been on this journey for AI for quite some time now. I started to use machine learning, I think it was in 2017 or ’18, to forecast demand. And I think I was one of the first to publish about that. Because back in 2018, you didn’t have so much resources online that you could read on how to apply machine learning to forecast demand. And that’s how I ended up writing my first book, Data Science for Supply Chain Forecasting, where I was basically trying to explain to whoever wanted to read it, how can you make your own machine learning model to forecast demand? And I really did my best at trying to explain that the way I would’ve loved that someone explained it to me one year earlier.
Now, of course, the technology evolved over the years, and every single year what I see is machine learning is faster. Machine learning is easier to use. And it’s more accurate. Year after year, it’s getting better. Statistical tools, so the one that most of software vendors are using right now, they’re not evolving to put it simply, or maybe the improvement is a tenth of a person every year. So, basically, you cannot expect as a supply chain leader that statistical models are going to get better. And the statistical model you have today, you could have done the same 10 years or 15 years ago. It’s exactly the same, basically.
Now, with machine learning, again, every year, it’s getting better. And every year, it seems for everyone that, well, that’s the future. Now, why and how is it so much better? One of the things that machine learning is able to do, it’s able to understand very complicated patterns in demand. And it’s also extremely good at understanding the relationship between prices, promotion, shortages, pre-orders, inventory located at your client, historical demand, seasonality, discounts, all of that. You can mix all of that in machine learning, and machine learning makes sense out of it. As I said, I’m working on a retailer right now. You can also mix this with, “Where’s the condition?”, if you do short-term forecasting. You can mix this with opening hours of your stores. You can mix this with, “Is tomorrow going to be a Friday or Saturday? Is it some holiday? Or was it some holiday last week? Are we going to go on holiday next week?”
Machine learning is able to deal with so much features and business drivers that really do impact demand. So it’s really amazing to see that as soon as you have a business that is promotion driven, and historically you had so many shortages that they’re attacking the quality of your demand dataset, machine learning is able to make a lot of sense out of that. Statistical tool, I’m not saying that it’s impossible to make sense out of that, but that would be dramatically difficult.
Now, on top of that, and I would nearly say by default, well, if you know how to use it, machine learning is going to give you a 10% or 20% accuracy boost, forecasted or reduction to put it technically correctly, compared to statistical engine. So, basically, machine learning today, even if you have no data, the simple fact that you start using these models, as long as you know how to use them, you’re going to get an instant boost in terms of forecasting accuracy. And that’s just wonderful. And, again, the technology is growing, so what we get today, in five years, the spread between the two technologies is only going to get wider. And that’s quite exciting, I think.
Lucie Phillips:
Yeah, that was going to be my next question. What do you think the future holds for the industry with, well, the future potential of machine learning?
Nicolas Vandeput:
So, for me, there is no doubt that companies that are able today to just, I would just say, structure the data, in the sense that they can have a nice dataset of, “This is our historical orders, historical pre-orders. This is our marketing plan, promotion plan, for the next 12 months and for the last five years. We have access to all the transactions we did, with all the prices for every single transaction,” things like that. If today you are a supply chain, a business with such amount of data, there is no doubt that if you access the right machine learning capabilities, you can reduce your forecast error by 20% or 25%, compared to statistical engines. And you can do this very easily in just one month you have it, and it’s up and running. So definitely we will have more and more businesses who are able to gather and structure this data, and start leveraging that to generate this forecast. And they’re going to get an instant boost in accuracy.
Again, what’s very important to understand is, if you get better at predicting the future, and if you get 25% better at predicting the future, all the decisions, every single decision your team’s going to make about inventory, about sizing, a warehouse, about opening your plant, every single decision’s going to get better as well. And that’s, I think, amazing.
Lucie Phillips:
Yeah, that’s very exciting. Well, sadly, we are coming to the end of our time today, so I have one last question for you. And it’s, do you have any final piece of wisdom, or the advice you always give, for any business that’s striving to stay ahead in what is an increasingly competitive market? What’s your one nugget that you like to give to your customers?
Nicolas Vandeput:
I think, today, if you’re the leader of a demand planning process and S&OP process, if you don’t have already this forecast value added mindset that I just talked about, I think that would be your number one priority. Without this, there is… I say that, for me, I like to put it that this is like the foundation of demand planning excellence. If you don’t have forecast value added, there is no way we can measure the added value of, for example, using machine learning, of your team, of anything. So the first thing you have to do is implement forecast value added. That’s really my 101 advice for people.
Lucie Phillips:
Okay. Well, hopefully, all of our listeners will take that away with them and keep that locked in their brain for their future strategy planning. Which just brings me to the end of the podcast, and I want to say thank you so much, Nicolas, for your time and for your insights. It was really fascinating having this conversation with you today. And thank you so much to all of our listeners for joining us for another episode of Freight to the Point. Don’t forget to like and subscribe to our series on Spotify, Apple Podcasts, or Google Podcasts if you enjoyed it. And if you do have any questions or feedback, then please feel free to reach out to us on LinkedIn. Thank you very much. We’d love to hear from you again soon.
Nicolas Vandeput:
Perfect. Thank you so much, Lucie.
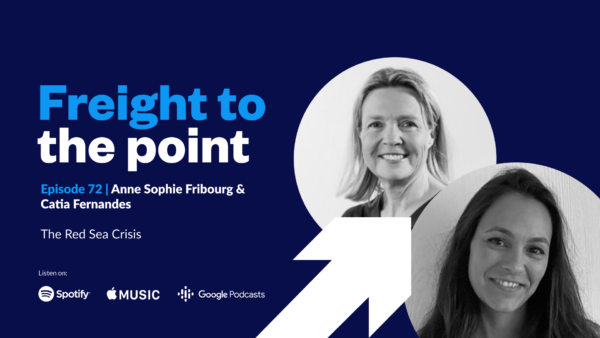
Episode 72: The Red Sea Crisis
In the latest episode of Freight to the Point, we’ve featured our most rec...
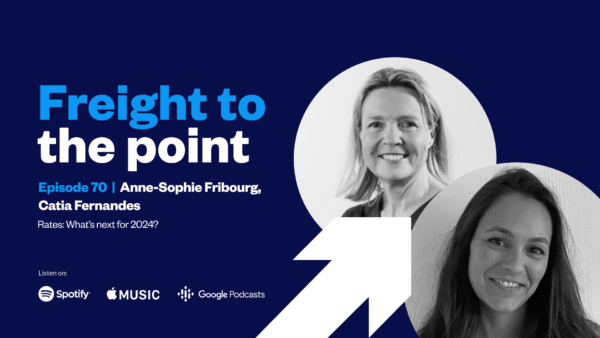
Episode 70: Rates: What's next for 2024?
As we prepare for the year ahead, it's crucial to consider the three pillars...
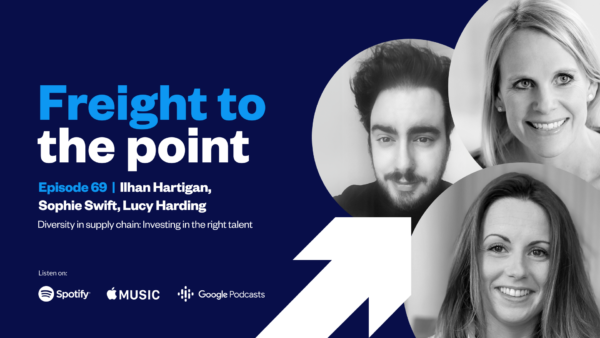
Episode 69: The Power of Diversity in the Supply Chain: Opportunities and Challenges
To make the right changes in an organisation, it's crucial to attract the ri...